Llama is a family of open large language models (LLMs) and large multimodal models (LMMs) from Meta. The latest version is Llama 4. It's basically the Facebook parent company's response to OpenAI and Google Gemini—but with one key difference: all the Llama models are freely available for almost anyone to use for research and commercial purposes.
That's a pretty big deal, and it has meant that the various Llama models have become incredibly popular with AI developers. Let's explore what Meta's "herd" of Llama models offers.
Table of contents:
What is Llama?
Llama is a family of LLMs (and LLMs with vision capabilities, or LMMs) like OpenAI's GPT and Google Gemini. Currently, the version numbers are a bit of a mess. Meta is at Llama 4 for some models, and Llama 3.3, 3.2, and 3.1 for others. As more of the Llama 4 herd is released, I suspect the various Llama 3 models will be culled—but they're still available and supported for now.
As I write this, the models available to download from Meta are:
Llama 3.1 8B
Llama 3.1 405B
Llama 3.2 1B
Llama 3.2 3B
Llama 3.2 11B-Vision
Llama 3.2 90B-Vision
Llama 3.3 70B
Llama 4 Scout
Llama 4 Maverick
There are also two unreleased Llama 4 models:
Llama 4 Behemoth
Broadly speaking, all the Llama models operate on the same underlying principles. They use variations on transformer architecture, and were developed using pre-training and fine-tuning. The biggest differences are that the Llama 4 models are natively multimodal and use a mixture-of-experts architecture (more on that in a bit).
When you enter a text prompt or provide a model with text input in some other way, it attempts to predict the most plausible follow-on text using its neural network—a cascading algorithm with billions of variables (called "parameters") that's modeled after the human brain. (A similar process happens with images for the models that support it.)
The various Llama 3 models offer different price-performance tradeoffs. For example, small models like Llama 3.1 8B and Llama 3.2 3B are designed to run on edge devices like smartphones and computers, or to be incredibly quick and cheap to operate on more powerful hardware. The largest model, Llama 3.1 405B, has the highest performance in most situations, but it requires the most resources to run. The Vision models are for multimodal use cases, and Llama 3.3 70B offers an excellent balance between performance and cost.
The two Llama 4 models—Llama 4 Scout and Llama 4 Maverick—use a slightly different approach to parameters called a mixture-of-experts (MoE). Llama 4 Scout has 109B total parameters but only uses 17B at once. Llama 4 Maverick has 400B total parameters, but also only uses a maximum of 17B. This approach allows AI models to be both more powerful and more efficient, though they're more complex to develop.
In addition to Scout and Maverick, Meta has announced Llama 4 Behemoth. It also uses the MoE architecture and has 2T total parameters with 288B active parameters. It's still in training.
One noticeable absence from the Llama 4 announcement is any kind of reasoning model. There's a teaser page, so it is coming soon, but for now, the Llama herd is limited to non-reasoning models.
Meta AI: How to try Llama
Meta AI, the AI assistant built into Facebook, Messenger, Instagram, and WhatsApp, now uses Llama 4—at least in the U.S. The best place to check it out is the dedicated web app.
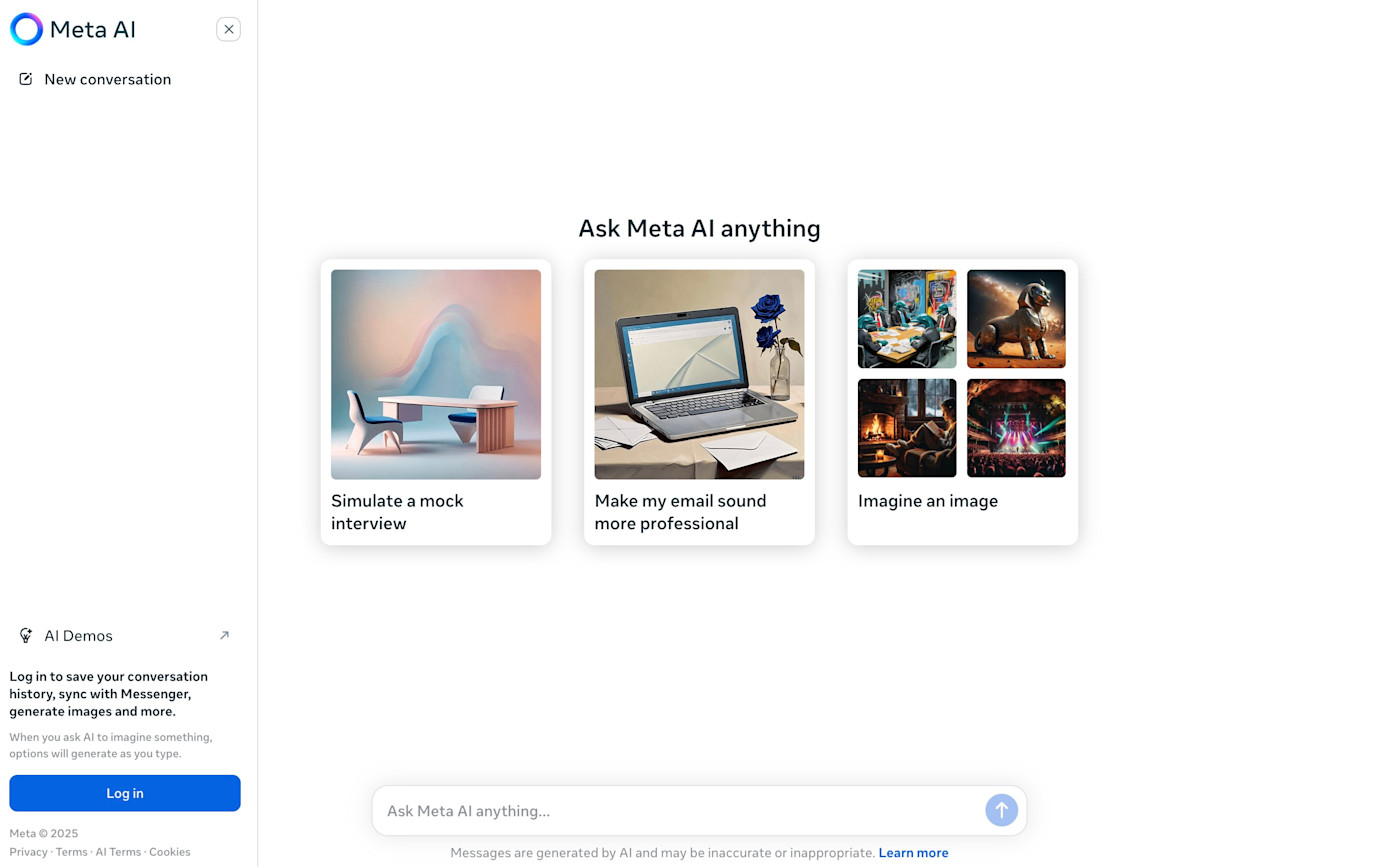
How does Llama 4 work?
Llama 4 uses a mixture-of-experts architecture. Scout has 109B parameters across 16 experts and activates 17B parameters at once. Maverick has 400B parameters across 128 experts and also activates 17B parameters at once.
Each expert is a subsystem that specializes in one particular area. While LLMs don't work with language the same way as humans, if you imagine that Scout has one expert that handles English literature, another that handles computer coding, and another that handles biology, you aren't too far off. Maverick, with its larger number of parameters and experts, has even more specific subsystems; instead of an expert in biology, it has something like an expert in microbiology and an expert in zoology.
The key is that when one of the Llama 4 models' MoE networks is activated, a gating network selects which of the experts is most appropriate to use alongside a shared expert that's used every time. (Imagine the shared expert handling general knowledge.) If you ask Scout a detailed question on apex predators, it will answer by activating its biology expert and shared expert. If you ask it for a treatise on Jaws, it will activate the English lit expert and the shared expert. This way, it only needs to activate 17B of its 109B parameters to generate a response.
On the other hand, if you send the same prompts to Llama 3.3 70B, all 70B parameters are activated every single time.
Of course, this vastly simplifies things. LLMs work using "tokens" that are mapped in multi-dimensional vector space. Each one is a word or semantic fragment that allows the model to assign meaning to text and plausibly predict follow-on text. If the words "Apple" and "iPhone" consistently appear together, it's able to understand that the two concepts are related—and are distinct from "apple," "banana," and "fruit"—because of how all the vectors are related. Each expert emerges while the model is being trained and takes a chunk of vector space, rather than a neatly delineated subject like biology, but the overarching idea still holds true.
To reach this point, the Llama 4 models were trained on trillions of tokens of text, as well as billions of images. Some of the data comes from publicly available sources like Common Crawl (an archive of billions of webpages), Wikipedia, and public domain books from Project Gutenberg, while some of it was also "synthetic data" generated by earlier AI models. (None of it is Meta user data.)
In addition to undergoing their own training, Scout and Maverick were also distilled from Behemoth, which Meta claims is "among the world's smartest LLMs." In essence, this means that Scout and Maverick were trained to match Behemoth's outputs, allowing them to better match its performance despite being significantly smaller models.
Of course, training an AI model on the open internet is a recipe for racism and other horrendous content, so Meta also employed other training strategies, like supervised fine-tuning, online reinforcement learning, and direct preference optimization. These all combine to steer the AI to generate useful and appropriate outputs.
All the Llama models are intended to be a base for developers to build from. If you want to create an LLM to generate article summaries in your company's particular brand style or voice, you can train Llama models with dozens, hundreds, or even thousands of examples and create one that does just that. Similarly, you can configure one of the models to respond to your customer support requests by providing it with your FAQs and other relevant information like chat logs. Or you can just take any Llama model and retrain it to create your own completely independent LLM.
Llama vs. GPT, Gemini, and other AI models: How do they compare?
Llama 4 Maverick and Scout are solid open models, though they don't offer best-in-class performance. In particular, the lack of a reasoning model (so far) keeps them from the top of most benchmarks.

Llama 4 Maverick is competitive with DeepSeek V3, Grok 3, GPT-4o, Claude Sonnet 3.7, and Gemini 2.0 Flash. As you can see in the chart above from Artificial Analysis, it's a decent non-reasoning model, though its key advantage is that it's the highest performing open multimodal model—and the highest performing non-Chinese open language model.
Maverick's MoE structure should also make it cost-efficient to run, especially when compared to proprietary models like GPT-4o. An experimental version is currently second in the chatbot arena, so it's certainly showing some promise. It has a context window of one million tokens, which is good, but matched by other models.
Llama 4 Scout is competitive with GPT-4o mini, but it's interesting for two reasons. First, it's designed to operate on a single H100 GPU. While this is still a server class GPU, larger models typically operate on a cluster of multiple GPUs rather than a single dedicated GPUs. Second, it has a context window of 10 million tokens, which really is best in class. The caveat is that no current provider is set up to offer it yet.
While Meta has released some provisional performance scores for Behemoth—it apparently beats GPT-4.5 on a few benchmarks—things move so fast in the AI space that it's not worth dwelling on them too much until it's available. Similarly, any Llama 4 reasoning model is going to be a big deal.
Llama 4 is obviously the future of the Llama herd, but the Llama 3 models remain good options. They can no longer be said to offer state-of-the-art performance, but they can be affordable and effective.
Why Llama matters
Most of the LLMs you've heard of—OpenAI's o1 and GPT-4o, Google's Gemini, Anthropic's Claude—are all proprietary and closed source. Researchers and businesses can use the official APIs to access them and even fine-tune versions of their models so they give tailored responses, but they can't really get their hands dirty or understand what's going on inside.
With Llama, though, you can download the model right now, and as long as you have the technical chops, get it running on a cloud server or just dig into its code. You can run Llama 3 models on some computers, though Llama 4 Scout and Maverick are too large large for home use.
And much more usefully, you can also get it running on Microsoft Azure, Google Cloud, Amazon Web Services, and other cloud infrastructures so you can operate your own LLM-powered app or train it on your own data to generate the kind of text you need. Just be sure to check out Meta's guide to responsibly using Llama—the license isn't quite as permissive as a traditional open source license.
Still, by continuing to be so open with Llama, Meta is making it significantly easier for other companies to develop AI-powered applications that they have more control over—as long as they stick to the acceptable use policy. Concerningly, users in the EU are currently banned from Llama 4, but we'll see if that changes as it rolls out. The only other big limits to the license are that companies with more than 700 million monthly users have to ask for special permission to use Llama, so the likes of Apple, Google, and Amazon have to develop their own LLMs.
In a letter that accompanied Llama 3.1's release, CEO Mark Zuckerberg was incredibly transparent about Meta's plans to keep Llama open:
"I believe that open source is necessary for a positive AI future. AI has more potential than any other modern technology to increase human productivity, creativity, and quality of life—and to accelerate economic growth while unlocking progress in medical and scientific research. Open source will ensure that more people around the world have access to the benefits and opportunities of AI, that power isn't concentrated in the hands of a small number of companies, and that the technology can be deployed more evenly and safely across society."
And really, that's quite exciting—as long as the EU thing gets sorted. Sure, Meta will benefit by being somewhat in the driving seat of one of the most important AI models. But independent developers, companies that don't want to be locked into a closed system, and everyone else interested in AI will benefit. So many of the big developments in computing over the past 70 years have been built on top of open research and experimentation, and now AI seems to be one of them. While Google, OpenAI, and Anthropic are always going to be players in the space, they won't be able to build the kind of commercial moat or consumer lock-in that Google has in search and advertising.
By letting Llama out into the world, there will likely always be a credible alternative to closed source AIs.
Related reading:
This article was originally published in August 2023. The most recent update was in April 2025.